Program Schedule
Monday, June 26
8:00 a.m. – 9:00 a.m. Breakfast & Opening remarks FGH Atrium
9:00 a.m. – 10:00 a.m. Keynote FGH 134 - Lecture Hall
Smart Technologies for Smarter Buildings
Philip V. Orlik (Mitsubishi Electric Research Laboratories, USA)
10:00 a.m. – 10:30 a.m. BREAK FGH Atrium
10:30 a.m. – 12:00 p.m. Concurrent Workshops
7th International Workshop on Big Data and FGH 110
IoT Security in Smart Computing (BITS 2023)
8th IEEE Workshop on Smart Service Systems (SmartSys) FGH 138
9th Workshop on Sensors and Smart Cities (SSC) FGH 132
12:00 p.m. – 1:30 p.m. LUNCH FGH Atrium
1:30 p.m. – 3:00 p.m. Concurrent Workshops
International Workshop on Smart Agriculture FGH 110
for the Environmental Emergency (SmartAgr)
8th IEEE Workshop on Smart Service Systems (SmartSys) FGH 138
9th Workshop on Sensors and Smart Cities (SSC) FGH 132
3:00 p.m. – 3:30 p.m. BREAK FGH Atrium
3:30 p.m. – 5:00 p.m. SESSION 1 FGH 134 - Lecture Hall
Smart Applications
5:00 p.m. – 5:30 p.m. BREAK FGH Atrium
5:30 p.m. – 7:00 p.m. Panel I FGH 134 - Lecture Hall
Trustworthy and Explainable AI for Smart Computing
7:00 p.m. SELF-ORGANIZED SOCIAL ACTIVITY
Suggestions will be provided on the conference website
7:00 p.m. Organizing and Technical Program Emery Nashville
Committee Dinner
Tuesday, June 27
8:00 a.m. – 9:00 a.m. Breakfast & Opening remarks FGH Atrium
9:00 a.m. – 10:00 a.m. Keynote FGH 134 - Lecture Hall
Smart Mobility Services on the Edge
Liam Pedersen
(Renault-Nissan-Mitsubishi Alliance Innovation Lab, USA)
10:00 a.m. – 10:30 a.m. BREAK FGH Atrium
10:30 a.m. – 12:00 p.m. SESSION 2 FGH 134 - Lecture Hall
Machine Learning for Sensing
12:00 p.m. – 1:00 p.m. LUNCH FGH Atrium
1:00 p.m. – 3:00 p.m. Concurrent Tutorials
From Quantum Computing to Quantum Networks FGH 136
Algorithms for Optimizing Public Transit Systems FGH 138
3:00 p.m. – 3:30 p.m. BREAK FGH Atrium
3:30 p.m. – 4:30 p.m. SESSION 3 FGH 134 - Lecture Hall
Middleware for Smart Computing
4:30 p.m. – 5:00 p.m. BREAK FGH Atrium
Move to the Demo and Poster Session
5:00 p.m. – 7:30 p.m. Demo / Poster Session with Reception Engineering Science Building
Wednesday, June 28
8:00 a.m. – 9:00 a.m. Breakfast & Opening remarks FGH Atrium
9:00 a.m. – 10:00 a.m. Keynote FGH 134 - Lecture Hall
Reducing the complexity, latency and energy of Inference
Execution for edge-based Deep Neural Network Models
Archan Misra (Singapore Management University, Singapore)
10:00 a.m. – 10:30 a.m. BREAK FGH Atrium
10:30 a.m. – 12:00 p.m. SESSION 4 FGH 134 - Lecture Hall
Computer Vision for Smart Computing
12:00 p.m. – 1:30 p.m. LUNCH FGH Atrium
1:30 p.m. – 3:00 p.m. Panel II FGH 134 - Lecture Hall
Reports from the Field: Deploying Smart Computing
Technology to Meet Diverse Community Needs
3:00 p.m. – 3:30 p.m. BREAK FGH Atrium
3:30 p.m. – 4:30 p.m. SESSION 5 FGH 134 - Lecture Hall
Networked and Distributed Smart Computing
4:30 p.m. – 5:00 p.m. WIP PRESENTATIONS FGH 134 - Lecture Hall
5:00 p.m. – 6:00 p.m. BREAK
Travel to Banquet
6:00 p.m. – 8:30 p.m. CONFERENCE BANQUET Country Music Hall of Fame
Thursday, June 29
8:00 a.m. – 9:00 a.m. Breakfast & Opening remarks FGH Atrium
9:00 a.m. – 10:00 a.m. Keynote FGH 134 - Lecture Hall
Enabling Climate Smart Agriculture through Smart Computing
Baskar Ganapathysubramanian (Iowa State University, USA)
10:00 a.m. – 10:30 a.m. BREAK FGH Atrium
10:30 a.m. – 12:00 p.m. SESSION 6 FGH 134 - Lecture Hall
Machine Learning for Control
12:00 p.m. – 1:30 p.m. LUNCH FGH Atrium
1:30 p.m. – 3:00 p.m. N2Women FGH 138
Inclusive Excellence - What does that actually mean?
3:00 p.m. – 3:30 p.m. BREAK FGH Atrium
3:30 p.m. – 5:00 p.m. PhD FORUM FGH 110
5:00 p.m. Adjourn
Keynotes
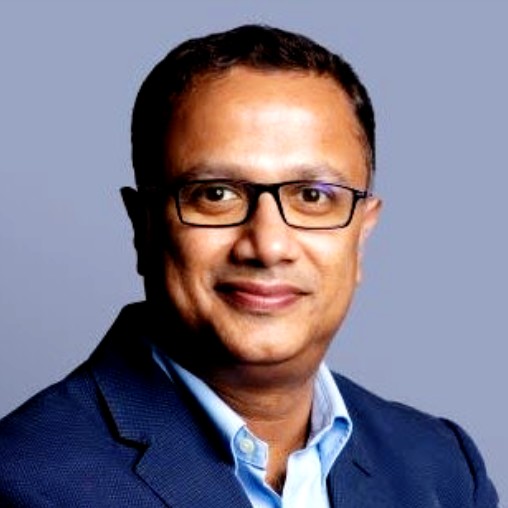
Archan Misra
Vice Provost (Research) and Professor of Computer Science at Singapore Management University (SMU)Reducing the complexity, latency and energy of Inference Execution for edge-based Deep Neural Network Models
Abstract: Several embodiments of smart computing applications require real-time, on-device perception and response in immersive field environments. Optimizing the execution of heavyweight DNN models for such perception tasks on resource-constrained edge devices remains a formidable challenge. Using two exemplar applications, vehicular traffic tracking and human-robot interactive task execution, I shall describe two powerful and innovative approaches to reduce the complexity, latency and energy of such edge-based DNN inference execution. The first involves the notion of criticality-aware spatiotemporal prioritization of selected regions from multiple video sensor streams, allowing an edge device to achieve 5-8x increase in DNN processing throughput with practically no loss in object detection and tracking accuracy. The second involves the use of "soft-skipping", a dynamic DNN selective execution technique to support low-latency multi-modal human instruction comprehension (combining visual, verbal and gestural input) for natural human-robot interaction. I shall conclude with an overview of open challenges and research directions in the broad area of interactive human-machine task execution.
Archan Misra is Vice Provost (Research) and Professor of Computer Science at Singapore Management University (SMU). Archan has provided leadership to a number of multi-million dollar, large-scale research initiatives at SMU, including the LiveLabs, LARC and CASA research centers, which collectively developed innovative mobile/wearable computing technologies for urban computing applications. He is a current recipient of the prestigious Investigator grant (from Singapore's National Research Foundation) for sustainable human-machine interaction intelligence. His current research interests lie in ultra-low energy execution of machine perception algorithms on IoT and edge devices to support advances in natural human-machine interaction. Over a 25+ year research career spanning both academics and industry (at IBM Research and Bellcore), Archan has published on, and practically deployed, technologies spanning wireless networking, mobile & wearable sensing and urban mobility analytics. An ACM Distinguished Member, Archan holds a Ph.D. from the University of Maryland at College Park, and chaired the IEEE Computer Society's Technical Committee on Computer Communications (TCCC) from 2005-2007.In 2017, Dr. Ploetz joined the School of Interactive Computing at the Georgia Institute of Technology in Atlanta, USA where he works as an Associate Professor of Computing. Prior to this he was an academic at the School of Computing Science at Newcastle University in Newcastle upon Tyne, UK, where he was a Reader (Assoc. Prof.) for "Computational Behaviour Analysis" affiliated with Open Lab, Newcastle's interdisciplinary research centre for cross-disciplinary research in digital technologies.
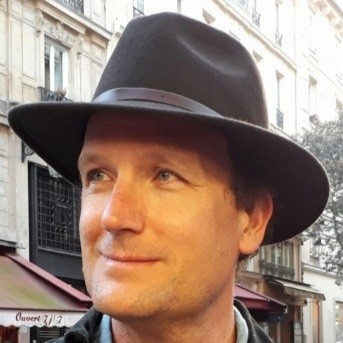
Liam Pedersen
Chief Scientist at Renault-Nissan-Mitsubishi Alliance Innovation LabSmart mobility services on the edge
Abstract Connectivity, smart infrastructure and autonomous driving software hosted on the edge computing will transform the ways in which we use and drive cars, the freeway system and the electrical grid. In this talk I will present examples of smart cloud based services for managing freeway congestion, low cost autonomous valet parking and EV integration with the grid.
Liam Pedersen is Chief Scientist at the Renault-Nissan-Mitsubishi Alliance Innovation Lab - Silicon Valley, where he works on autonomous driving and urban mobility. Prior to this he worked on robotic systems for planetary exploration at NASA’s Ames Research Center in California. He holds a Ph.D. in robotics from Carnegie Mellon University (for the robotic discovery of a meteorite), and is the recipient of NASA’s Public Service Medal and the NSF Antarctic Service Medal.
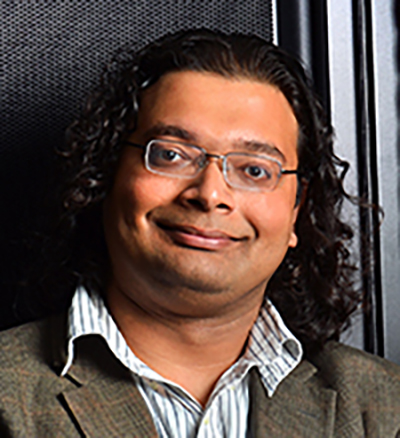
Baskar Ganapathysubramanian
Joseph C. and Elizabeth A. Anderlik Professor in Engineering and Professor, Mechanical Engineering, Iowa State UniversityEnabling climate smart agriculture through smart computing
Abstract Advances in AI and machine learning concepts are transforming the way modern agriculture is practiced. The desire for profitability, productivity, and sustainability in agriculture provides a number of opportunities for smart computing, for example the interplay of data collection, model training, deployment, decision support and feedback. I will present a few examples involving data annotation efficient training of ML models for pest and disease detection, context aware machine learning models for trait extraction and decision support, as well as extraction of 3D structure of plants and fields. These examples illustrate collaborative work with a group of colleagues within two large projects -- AI Institute for Resilient Agriculture (AIIRA), and Context-Aware Learning for Sustainable Cyber-agricultural Systems (COALESCE).
Baskar Ganapathysubramanian Baskar Ganapathysubramanian is Anderlik Professor of Engineering at Iowa State University. Baskar received his BTech from IIT Madras, and a PhD from Cornell University. He directs a curiosity driven, computational sustainability group (me.iastate.edu/bglab) with research interests in the areas of scientific computing, applied mathematics, and machine learning with applications in food, energy, and healthcare systems. He is the director of the NSF/USDA funded AI Institute for Resilient Agriculture (aiira.iastate.edu) which is a multi-institutional project (Iowa State, Carnegie Mellon, NYU, U Nebraska, U Arizona) focused on use-inspired AI developments for agriculture. He is associate director of the Translational AI Center (trac-ai.iastate.edu) at Iowa State University which seeks to advance use-inspired AI developments.
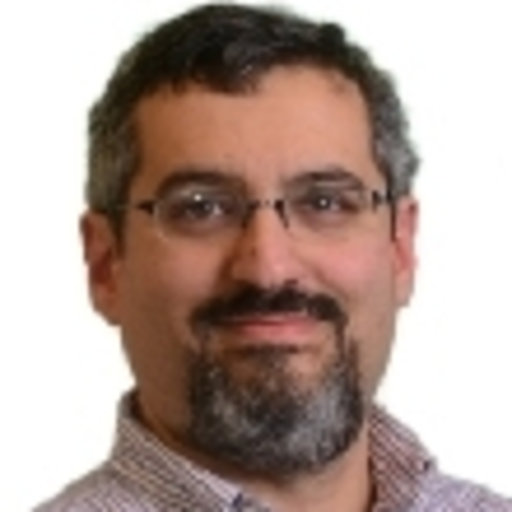
Philip V. Orlik
Vice President and Director at Mitsubishi Electric Research LaboratoriesSmart Technologies for Smarter Buildings
Abstract Mitsubishi Electric Corporation recognizes the need to reduce carbon emissions and has set ambitious goals to continually reduce emissions and achieve net-zero emissions by 2050. Achieving these goals requires changes in policy, management as well as technical innovation throughout the company’s products and operations. As a representative of corporate research and development I would like to discuss how these goals and vision for a sustainable future together with advances in sensing, IoT and computing are driving the research agenda in industry. In this talk I will focus on the domain of smart buildings and discuss some of the research challenges and opportunities that arise as we seek to achieve net-zero emissions. Smart building environments provide ample opportunity to apply IoT and advanced computing/learning methods to solve a variety of complex problems. I will touch on examples such as, calibrating building models with thousands of parameters, understanding and controlling airflow, predicting occupant motion and comfort as well as room occupancy and controlling building systems to ensure the most efficient use of energy.
Philip V. Orlik (M’97-11, SM’12) was born in New York, NY in 1972. He received the B.E. degree in 1994 and the M.S. degree in 1997 both from the State University of New York at Stony Brook. In 1999 he earned his Ph. D. in electrical engineering also from SUNY Stony Brook. Since 2000 he has been with Mitsubishi Electric Research Laboratories Inc. located in Cambridge, MA where he is currently Vice President and Research Director responsible for research in the areas of signal processing, data analytics, robotics, and electronic devices. His primary research focus is on advanced wireless and wired communications as well as sensor/IoT networks. He has been a contributor to various IEEE 802 standards including 802.11n and 802.15.4a where he was also a technical editor of the UWB physical layer and was network layer technical editor for the initial version of ZigBeeTM. His other research interests include vehicular/car-to-car communications, mobility modeling, network performance analysis, and queuing theory.
Tutorials
From quantum computing to quantum networks
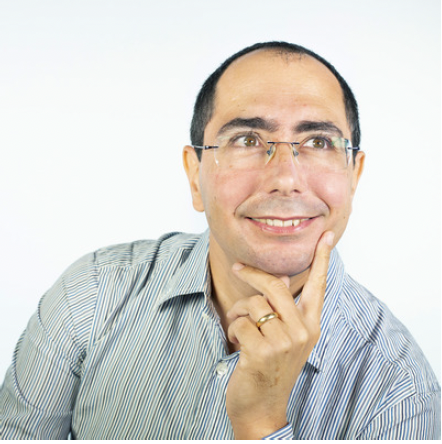
Claudio Cicconetti IIT-CNR
Abstract Thanks to recent advances of technologies for the manipulation of matter at very small scale, quantum technologies are experiencing a significant boost of public and private investments, as well as raising interest in the scientific community. Quantum computing addresses the construction and operation of quantum computers to solve more efficiently instances of specific problems that are difficult to tackle with classical computers. On the other hand, quantum communications cover the transmission of quantum states across distances.
After being awarded a PhD in Information Engineering from the University of Pisa (Italy) and following an experience as a postdoc researcher in the same university, Claudio Cicconetti has been working in Intecs S.p.a. (Italy) from 2009 to 2013 as an R&D manager and in MBI S.r.l. (Italy) from 2014 to 2018 as a principal software engineer. He is now a senior researcher in the Ubiquitous Internet group of IIT-CNR (Italy). He has been involved in several international R&D projects funded by the European Commission and the European Space Agency. He serves as a member of the TPC of several international conferences (IEEE PerCom, IEEE SMARTCOMP, IEEE WoWMoM) and he is an editor of the IEEE Networking Letters. He co-authored 60+ papers published in international journals and peer-reviewed conference proceedings and two international patents. His main research interests are serverless edge computing and quantum networking.
Algorithms for Optimizing Public Transit Systems
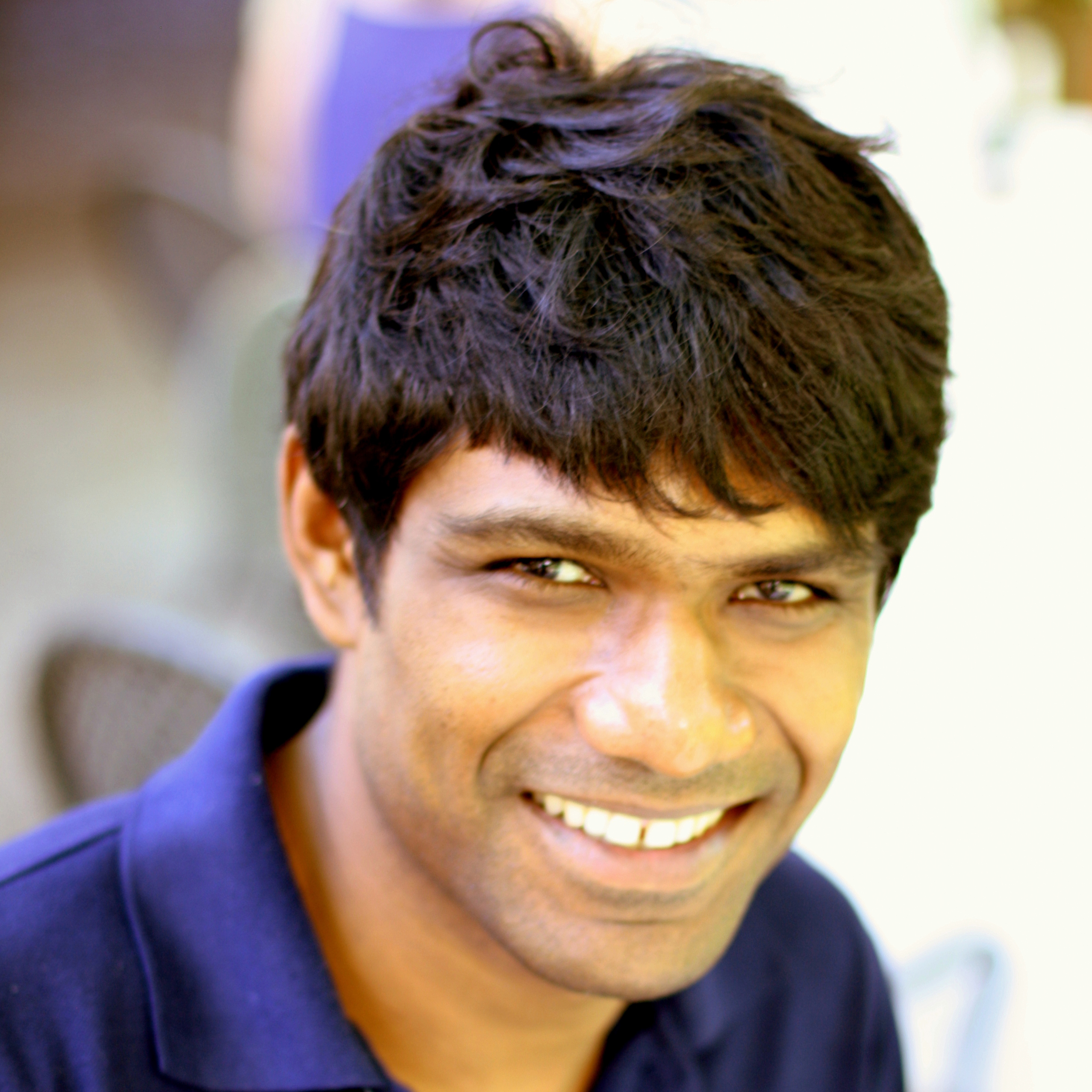
Samitha Samaranayake, Cornell
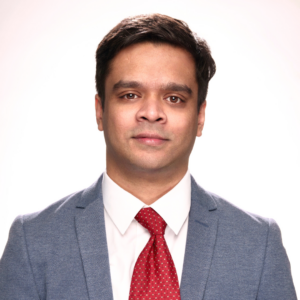
Ayan Mukhopadhyay, Vanderbilt
Abstract A well-functioning public transit system fosters the growth and expansion of businesses, distributes social and economic benefits, and links the capabilities of community members. Transit as a service is especially important for low-income communities and individuals with disabilities (or short-term issues) as they often do not own (or are temporarily unable to use) private vehicles and must rely on public transit for connecting to employment opportunities, education, healthcare, and other essential services. Further, as we face an alarming climate crisis, reducing personal vehicle usage and prioritizing efficient public transit promises a more sustainable future. There are more than 7000 public transit agencies in the U.S., which collectively serve more than 60 billion passenger miles. However, many transit agencies struggle to meet their mission due to decreasing ridership, increasing operational costs, and competition from commercial providers. This tutorial will explore fundamental algorithmic and data-driven approaches to optimize transportation networks. We will discuss prior work on optimizing ridership, coverage, energy consumption, and equity and explore how the future of public transit will shape the future of our smart cities. We will also share open-source data curated by our team that can serve as a benchmark for optimizing some transit optimization problems.
Samitha Samaranayake is an Assistant Professor in the School of Civil and Environmental Engineering and a Graduate Field Faculty in the School of Operations Research and Information Engineering, the Center for Applied Math, and the Systems Engineering Program at Cornell. Before joining Cornell, he was a Postdoctoral Associate in the Laboratory for Information and Decision Systems (LIDS) at the Massachusetts Institute of Technology (MIT) and a contributing participant in the Institute for Pure and Applied Mathematics long program on New Directions in Mathematical Approaches for Traffic Flow Management in Fall 2015. Samitha holds a Ph.D. in Systems Engineering from the University of California, Berkeley.
Ayan Mukhopadhyay is a research scientist at Vanderbilt University, USA. His research interests include multi-agent systems, robust machine learning, and decision-making under uncertainty for social impact. He is the recipient of the Google AI Impact Scholar Award 2021. Before this, he was a Post-Doctoral Research Fellow at the Stanford Intelligent Systems Lab at Stanford University. Ayan holds a Ph.D. in Computer Science from Vanderbilt University.
Panels
Panel I
Trustworthy and Explainable Intelligent Systems for Smart City Services
Intelligent data-driven systems combe advances in sensing technologies, IoT and AI. They have shown great promise in transforming smart city applications. In healthcare, their potential benefits range from streamlining collaboration across healthcare professionals, to accelerating patient flow and improving overall patient and staff experience. In blue light applications, smart sensor systems have huge potential to enhance safety and increase situation awareness for emergency responders. In transport, mobile autonomy systems could revolutionize our approaches to streamlining traffic, reducing transit times and enhancing transport safety. Although such advances are technologically possible, they are still very slow to adopt in our cities. With experts from different public services and academia, this panel will explore key adoption barriers and opportunities, and will discuss the importance of making smart systems more trustworthy and explainable, as well as easy to connect with each other, and blend seamlessly within existing operations and processes.
Moderator:
Ayan Mukhopadhyay (Vanderbilt University, USA)Panelists
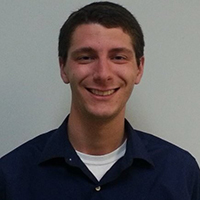
Dan Freudberg
Deputy Chief Operating Officer – Operations SystemsWeGo Public Transit
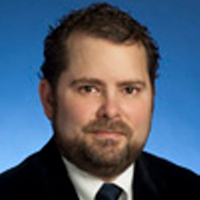
Brad Freeze
Deputy DirectorNashville Department of Transportation and Multimodal Infrastructure
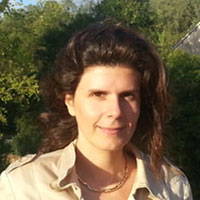
Niki Trigoni
Professor of Computing ScienceOxford University
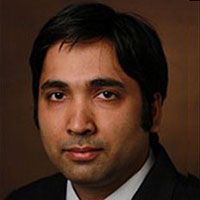
Abhishek Dubey
Associate Professor of Computer Science and Electrical and Computer EngineeringVanderbilt University
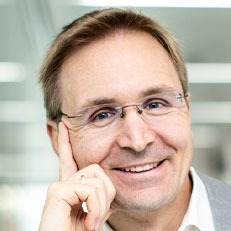
Stephan Sigg
Associate professor, Department of Communications and NetworkingAalto University, Finland
Panel II
Reports from the Field: Deploying Smart Computing Technology to Meet Diverse Community Needs
There is a growing demand for smart computing technologies to meet a range of community needs. This includes sensing systems, Internet of Things devices, cyber-physical systems, artificial intelligence applications, and more, to inform decision-making and enhance autonomy. A range of smart computing investments, both publicly and privately funded, are underway across the globe. These initiatives seek to address community challenges related to energy and resource usage, transportation and mobility, climate change, health and wellbeing, digital literacy, and more.
While demand for these technologies continues to grow, there are significant technical and societal challenges on the pathway from laboratory to real-world deployment. As a result, experiences vary widely from one application/community to another. This panel brings together academic researchers, agency officials, and municipal leaders from both the U.S. and the E.U. to share field experiences deploying smart computing to meet diverse community needs. The panel represents an important step in codifying a set of best practices for translating these technologies from the laboratory to the public sphere.
Moderator:
Dr. Jason Hallstrom (Florida Atlantic University and National Science Foundation, USA)Dr. Dirk Pesch (University of College Cork, Ireland)
Panelists
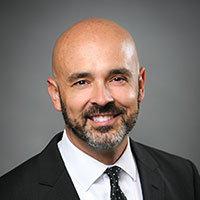
Keith Durbin
Chief Information OfficerMetropolitan Government of Nashville and Davidson County
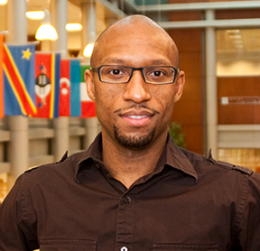
James Hill
Associate Professor, School of ScienceIndiana University – Purdue University Indianapolis
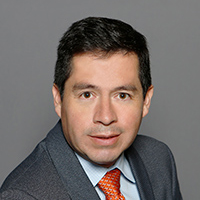
Oscar A. Mondragon Campos
Clinical Associate Professor, Computer ScienceThe University of Texas at El Paso
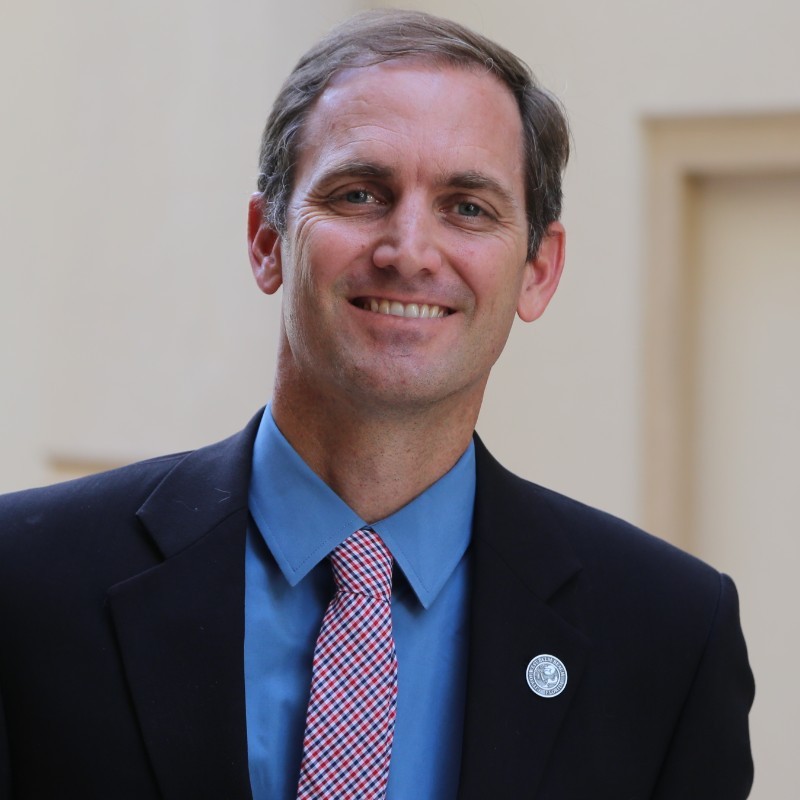
Christopher Roog
Executive Director, Community Redevelopment AgencyCity of West Palm Beach
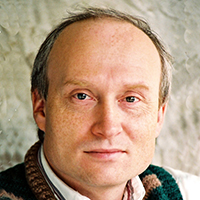
Oleg Sokolsky
Professor, Computer and Information ScienceUniversity of Pennsylvania
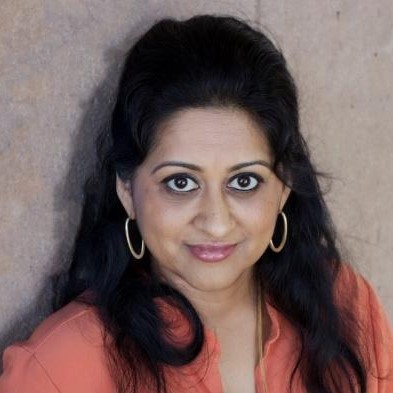
Nalini Venkatasubramaniam
Professor, School of Information and Computer SciencesUniversity of California, Irvine
N2 Women Event: Inclusive Excellence - What does it actually mean?
Abstract: In recent years, the concept “Inclusive Excellence” has gained popularity in academia, serving as a framework for promoting equity, diversity, and belonging. While the term holds promise, there is a concern that, like other well-intentioned diversity language, it may lose its significance without tangible implementation. This N2Women panel will delve into the experiences of female leaders, exploring their insights on how to effectively translate values into meaningful practices. The panel will foster a discussion on the practical strategies and actions that researchers can undertake to uphold the principles of inclusive excellence. By exploring the multifaceted nature of the buzzword, the panel aims to empower researchers with actionable insights to promote diversity and develop a sense of belonging within academic communities. Narrowing the gap between rhetoric and action, researchers will explore how to ensure the concept of inclusive excellence becomes a lived reality in academia.
Panelists
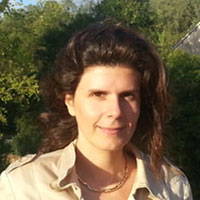
Dr. Niki Trigoni
ProfessorOxford University Department of Computer Science
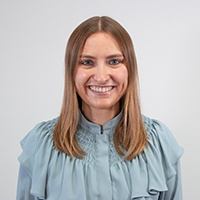
Dr. Francesca Meneghell
Assistant Professor in TelecommunicationsDepartment of Information Engineering at the University of Padova (Italy)
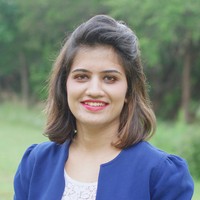
Dr. Heena Rathore
Assistant ProfessorDepartment of Computer Science at Texas State University, San Marcos, Texas, USA
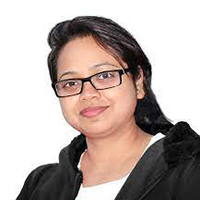
Dr. Priyanka Verma
Marie Skłodowska-Curie Postdoctoral Research FellowUniversity of Galway, Ireland
Workshops
In addition to the keynotes, panels, tutorials and main conference paper presentations, the conference includes four co-located workshops.
7th International Workshop on Big Data and IoT Security in Smart Computing (BITS 2023)
General Chairs
Program Co-Chairs
9th Workshop on Sensors and Smart Cities (SSC)
General Chairs
Program Co-Chairs
8th IEEE Workshop on Smart Service Systems (SmartSys)
General Chairs
Program Co-Chairs
International Workshop on Smart Agriculture for the environmental emergency (SmartAgr)
General Chairs
Main Technical Sessions
Session 1: Smart Applications
Monday, 15:30 - 17:00
Session Chair: Franca Delmastro (IIT-CNR, Italy)
CA-Wav2Lip: Coordinate Attention-based Speech to Lip Synthesis in the Wild
Authors: Kuan-Chieh Wang, Jie Zhang and Jingquan Huang (National Central University, Taiwan); Qi Li (Auburn University, USA); Min-Te Sun (National Central University, Taiwan); Kazuya Sakai (Tokyo Metropolitan University, Japan); Wei-Shinn Ku (Auburn University, USA)
AbstractWith the growing consumption of online visual contents, there is an urgent need for video translation in order to reach a wider audience from around the world. However, the materials after direct translation and dubbing are unable to create a natural audio-visual experience since the translated speech and lip movement are often out of sync. To improve viewing experience, an accurate automatic lip-movement synchronization generation system is necessary. To improve the accuracy and visual quality of speech to lip generation, this research proposes two techniques: Embedding Attention Mechanisms in Convolution Layers and Deploying SSIM as Loss Function in Visual Quality Discriminator. The proposed system as well as several other ones are tested on three audio-visual datasets. The results show that our proposed methods achieve superior performance over the state-of-the-art speech to lip synthesis on not only the accuracy but also the visual quality of audio-lip synchronization generation.
Nisshash: Design of An IOT-based Smart T-Shirt for Guided Breathing Exercises
Authors: Md Abdullah Al Rumon, Suparna Veeturi, Mehmet Seckin, Dhaval Solanki and Kunal Mankodiya (University of Rhode Island, USA)
AbstractBreathing exercises are gaining attention in managing anxiety and stress in daily life. Diaphragmatic breathing, in particular, fosters tranquility for both body and mind. Existing use cases, such as meditation, yoga, and medical devices for guided breathing, often require expert guidance, complex instruments, cumbersome devices, and sticky electrodes. To address these challenges, we present Nisshash, an IoT-based smart T-shirt offering a personalized solution for regulated breathing exercises. Nisshash is embedded with three-channel e-textile respiration sensors and a tailored analog front-end (AFE) board to simultaneously monitor respiration rate (RR) and heart rate (HR). In this work, we seamlessly integrate soft textile sensors into a T-shirt and develop a detachable and Wi-Fi-enabled (2.4GHz) bio-instrumentation board, creating a pervasive wireless system (WPS) for guided breathing exercises (GBE). The system features an intuitive graphical user interface (GUI) and a seamless IoT-based control and computing system (CCS). It offers realtime instructions for inhaling and exhaling at various breathing speeds, including slow, normal, and fast breathing. Functions such as filtering, peak detections for respiration, and heart rate analysis are computed conjointly at the sender and receiver ends. We utilized the Pan-Tompkins and custom algorithms to calculate HR and RR from the filtered time-series signals. We conducted a study with 10 healthy adult participants who wore the Tshirt and performed guided breathing exercises. The average respiration event (inhale-exhale) detection accuracy was ≈ 98%. We validated the recorded HR against the 3-lead PC-80B ECG monitoring device, achieving an accuracy of ≈ 99%. The RRHR correlation analysis showed an R square value of 0.987. Collectively, these results demonstrate Nisshash's potential as a personal guided breathing exercise solution.
Addressing APC Data Sparsity in Predicting Occupancy and Delay of Transit Buses: A Multitask Learning Approach
Authors: Ammar Bin Zulqarnain, Samir Amitkumar Gupta and Jose Talusan (Vanderbilt University, USA); Philip Pugliese (Chattanooga Area Regional Transportation Authority, USA); Ayan Mukhopadhyay and Abhishek Dubey (Vanderbilt University, USA)
AbstractPublic transit is a vital mode of transportation in urban areas, and its efficiency is crucial for the daily commute of millions of people. To improve the reliability and predictability of transit systems, researchers have developed separate single-task learning models to predict the occupancy and delay of buses at the stop or route level. However, these models provide a narrow view of delay and occupancy at each stop and do not account for the correlation between the two. We propose a novel approach that leverages broader generalizable patterns governing delay and occupancy for improved prediction. We introduce a multitask learning toolchain that takes into account General Transit Feed Specification feeds, Automatic Passenger Counter data, and contextual information temporal and spatial information. The toolchain predicts transit delay and occupancy at the stop level, improving the accuracy of the predictions of these two features of a trip given sparse and noisy data. We also show that our toolchain can adapt to fewer samples of new transit data once it has been trained on previous routes/trips as compared to state-of-the-art methods. Finally, we use actual data from Chattanooga, Tennessee, to validate our approach. We compare our approach against the state-of-the-art methods and we show that treating occupancy and delay as related problems improves the accuracy of the predictions. We show that our approach improves delay prediction significantly by as much as 6% in F1 scores while producing equivalent or better results for occupancy.
SrPPG: Semi-Supervised Adversarial Learning for Remote Photoplethysmography with Noisy Data
Authors: Zahid Hasan (University of Maryland Baltimore County, USA); Abu Zaher Md Faridee (University of Maryland, Baltimore County & Amazon, USA); Masud Ahmed, Shibi Ayyanar and Nirmalya Roy (University of Maryland Baltimore County, USA)
AbstractRemote Photoplethysmography (rPPG) systems offer contactless, low-cost, and ubiquitous heart rate (HR) monitoring by leveraging the skin-tissue blood volumetric variation-induced reflection. However, collecting large-scale time-synchronized rPPG data is costly and impedes the development of generalized end-to-end deep learning (DL) rPPG models to perform under diverse scenarios. We formulate the rPPG estimation as a generative task of recovering time-series PPG from facial videos and propose SrPPG, a novel semi-supervised adversarial learning framework using heterogeneous, asynchronous, and noisy rPPG data. More specifically, we develop a novel encoder-decoder architecture, where rPPG features are learned from video in a self-supervised manner (encoder) to reconstruct the time-series PPG (decoder/generator) with physics-inspired novel temporal consistency regularization. The generated PPG is scrutinized against the real rPPG signals by a frequency-class conditioned discriminator, forming a generative adversarial network. Thus, SrPPG generates samples without point-wise supervision, alleviating the need for time-synchronized data collection. We experiment and validate SrPPG by amassing three public datasets in heterogeneous settings. SrPPG outperforms both supervised and self-supervised state-of-the-art methods in HR estimation across all datasets without any time-synchronous rPPG data. We also perform extensive experiments to study the optimal generative setting (architecture, joint optimization) and provide insight into the SrPPG behavior.
Session 2: Machine Learning for Sensing
Tuesday, 10:30-12:00
Session Chair: Francesca Meneghello (University of Padova, Italy)
Combining Public Human Activity Recognition Datasets to Mitigate Labeled Data Scarcity
Authors: Riccardo Presotto (University of Milan, Italy); Sannara EK (Grenoble University & Grenoble Computer Science Laboratory, France); Gabriele Civitarese (University of Milan, Italy); François Portet (Laboratory LIG, UMR CNRS/INPG/UJF 5217, Team GETALP, France); Philippe Lalanda (Grenoble University, France); Claudio Bettini (Università degli Studi di Milano, Italy)
AbstractThe use of supervised learning for Human Activity Recognition (HAR) on mobile devices leads to strong classification performances. Such an approach, however, requires large amounts of labeled data, both for the initial training of the models and for their customization on specific clients (whose data often differ greatly from the training data). This is actually impractical to obtain due to the costs, intrusiveness, and time-consuming nature of data annotation. Moreover, even with the help of a significant amount of labeled data, model deployment on heterogeneous clients faces difficulties in generalizing well on unseen data. Other domains, like Computer Vision or Natural Language Processing, have proposed the notion of pre-trained models, leveraging large corpora, to reduce the need for annotated data and better manage heterogeneity. This promising approach has not been implemented in the HAR domain so far because of the lack of public datasets of sufficient size. In this paper, we propose a novel strategy to combine publicly available datasets with the goal of learning a generalized HAR model that can be fine-tuned using a limited amount of labeled data on an unseen target domain. Our experimental evaluation, which includes experimenting with different state-of-the-art neural network architectures, shows that combining public datasets can significantly reduce the number of labeled samples required to achieve satisfactory performance on an unseen target domain.
Optimizing IoT-based Human Activity Recognition on Extreme Edge Devices
Authors: Angelo Trotta, Federico Montori, Giacomo Vallasciani, Luciano Bononi and Marco Di Felice (University of Bologna, Italy)
AbstractWearable Internet of Things (IoT) devices with inertial sensors can enable personalized and fine-grained Human Activity Recognition (HAR). While activity classification on the Extreme Edge (EE) can reduce latency and maximize user privacy, it must tackle the unique challenges posed by the constrained environment. Indeed, Deep Learning (DL) techniques may not be applicable, and data processing can become burdensome due to the lack of input systems. In this paper, we address those issues by proposing, implementing, and validating an EE-aware HAR system. Our system incorporates a feature selection mechanism to reduce the data dimensionality in input, and an unsupervised feature separation and classification technique based on Self-Organizing Maps (SOMs). We developed the system on an M5Stack IoT prototype board and implemented a new SOM library for the Arduino SDK. Experimental results on two HAR datasets show that our proposed solution is able to overcome other unsupervised approaches and achieve performance close to state-of-art DL techniques while generating a model small enough to fit the limited memory capabilities of EE devices.
μ-FF: On-Device Forward-Forward Training Algorithm for Microcontroller
Authors: Fabrizio De Vita and Rawan M. A. Nawaiseh (University of Messina, Italy); Dario Bruneo (Universita di Messina, Italy); Valeria Tomaselli, Marco Lattuada and Mirko Falchetto (STMicroelectronics, Italy)
AbstractOn-device training is becoming a novel way to deliver intelligence into low cost hardware e.g., Micro Controller Units (MCUs) for the realization of low power tailored applications. However, the training of deep learning models on embedded systems is a very challenging process mainly due to their low amount of memory, available energy, and computing power which significantly limit the complexity of the tasks that can be executed, thus making impossible the use of traditional training algorithms such as backpropagation (BP). During these years techniques such as weights compression and quantization have emerged as solutions, but they only address the inference phase. Forward-Forward (FF) is a novel training algorithm that has been recently proposed as a possible alternative to BP when the available resources are limited. This is achieved by training the layers of a neural network separately, thus reducing the amount of required energy and memory. In this paper, we propose a variation of the original FF which tackles the training process with a multivariate Ridge regression approach and adopts the Mean Squared Error (MSE) as loss function. Such an approach does not use BP and does not need to compute gradients, thus saving memory and computing resources to enable the on-device training directly on MCUs of the STM32 family. Experimental results conducted on the Fashion-MNIST dataset demonstrate the effectiveness of the proposed approach in terms of memory and accuracy.
A Systematic Study on Object Recognition Using Millimeter-wave Radar
Authors: Maloy Kumar Devnath (UMBC, USA); Avijoy Chakma and Mohammad Saeid Anwar (University of Maryland Baltimore County, USA); Emon Dey (University of Maryland, Baltimore County, USA); Zahid Hasan (University of Maryland Baltimore County, USA); Marc Conn and Biplab Pal (UMBC, USA); Nirmalya Roy (University of Maryland Baltimore County, USA)
AbstractMillimeter-wave (MMW) radar is becoming an essential sensing technology in smart environments due to its light and weather-independent sensing capability. Such capabilities have been widely explored and integrated with intelligent vehicle systems, often deployed in industry-grade MMW radars. However, industry-grade MMW radars are often expensive and difficult to attain for deployable community-purpose smart environment applications. On the other hand, commercially available MMW radars pose hidden underpinning challenges that are yet to be well investigated for tasks such as recognizing objects, and activities, real-time person tracking, object localization, etc. Such tasks are frequently accompanied by image and video data, which are relatively easy for an individual to obtain, interpret, and annotate. However, image and video data are light and weather-dependent, vulnerable to the occlusion effect, and inherently raise privacy concerns for individuals. It is crucial to investigate the performance of an alternative sensing mechanism where commercially available MMW radars can be a viable alternative to eradicate the dependencies and preserve privacy issues. Before championing MMW radar, several questions need to be answered regarding MMW radar's practical feasibility and performance under different operating environments. To answer the concerns, we have collected a dataset using commercially available MMW radar, Automotive mmWave Radar (AWR2944) from Texas Instruments, and reported the optimum experimental settings for object recognition performance using several deep learning algorithms in this study. Moreover, our robust data collection procedure allows us to systematically study and identify potential challenges in the object recognition task under a cross-ambience scenario. We have explored the potential approaches to overcome the underlying challenges and reported extensive experimental results.
Session 3: Middleware for Smart Computing
Tuesday, 15:30 - 16:30
Session Chair: Yusuf Sarwar Uddin (University of Missouri-Kansas City, USA)
Qkd@Edge: Online Admission Control of Edge Applications with QKD-secured Communication
Authors: Claudio Cicconetti, Marco Conti and Andrea Passarella (IIT-CNR, Italy)
AbstractQuantum Key Distribution (QKD) enables secure communications via the exchange of cryptographic keys exploiting the properties of quantum mechanics. Nowadays the related technology is mature enough for production systems, thus field deployments of QKD networks are expected to appear in the near future, starting from local/metropolitan settings, where edge computing is already a thriving reality. In this paper, we investigate the interplay between the resource allocation of resources in the QKD network and edge nodes, which creates unique research challenges. After modeling mathematically the problem, we propose practical online policies for admitting edge application requests, which also select the edge node for processing and the path in the QKD network. Our simulation results provide initial insights into this emerging topic and lead the way to upcoming studies on the subject.
TACSim: An Extendable Simulator for Task Allocation Mechanisms in CrowdSensing
Authors: Christine Bassem (Wellesley College, USA)
AbstractWith the increased popularity of Mobile Crowd Sensing (MCS), large volumes of sensing data can be collected by leveraging the sensing capabilities of the mobile devices carried by crowds already roaming in a mobility field. In participatory MCS, tasks are allocated to participants via some allocation mechanism, which are challenging in terms of their evaluation due to the lack of general-purpose, modular, and extendable simulators. Thus, forcing researchers to either launch their own testbeds or develop single-purpose simulators. In this paper, we present our design and implementation of an extendable simulator, namely TACSim, for the evaluation of task allocation mechanisms in a participatory MCS setting over realistic urban environments. TACSim is designed to accommodate realistic urban road networks, as well as spatio-temporal traces of sensing tasks and participant mobility. Moreover, it includes various built-in autonomous task allocation mechanisms, which can be extended by researchers to accommodate their own algorithms with minimal effort. We discuss the components and architecture of the simulator, and present a use-case of integrating existing autonomous task allocation mechanisms that further exemplifies the usability and extendability of the simulator.
AnB: Application-in-a-Box to rapidly deploy and self-optimize 5G app
Authors: Kunal Rao and Murugan Sankaradas (NEC Laboratories America Inc., USA); Giuseppe Coviello (NEC Laboratories America, Inc., USA); Ciro De Vita, Gennaro Mellone and Wang-Pin Hsiung (NEC Laboratories America Inc., USA); Srimat Chakradhar (NEC Research Labs, USA)
AbstractWe present Application in a Box (AnB) product concept aimed at simplifying the deployment and operation of remote 5G applications. AnB comes pre-configured with all necessary hardware and software components, including sensors like cameras, hardware and software components for a local 5G wireless network, and 5G-ready apps. Enterprises can easily download additional apps from an App Store. Setting up a 5G infrastructure and running applications on it is a significant challenge, but AnB is designed to make it fast, convenient, and easy, even for those without extensive knowledge of software, computers, wireless networks, or AI-based analytics. With AnB, customers only need to open the box, set up the sensors, turn on the 5G networking and edge computing devices, and start running their applications. Our system software automatically deploys and optimizes the pipeline of microservices in the application on a tiered computing infrastructure that includes device, edge, and cloud computing. Dynamic resource management, placement of critical tasks for low-latency response, and dynamic network bandwidth allocation for efficient 5G network usage are all automatically orchestrated. AnB offers cost savings, simplified setup and management, and increased reliability and security. We've implemented several real-world applications, such as collision prediction at busy traffic light intersections and remote construction site monitoring using video analytics. With AnB, deployment and optimization effort can be reduced from several months to just a few minutes. This is the first-of-its-kind approach to easing deployment effort and automating self-optimization of the application during system operation.
Session 4: Computer Vision for Smart Computing
Wednesday, 10:30 - 12:00
Session Chair: Hamed Tabkhivayghan (UNC Charlotte, USA)
Vision Transformer-based Real-Time Camouflaged Object detection System at Edge
Authors: Rohan Putatunda, Md Azim Khan, Aryya Gangopadhyay, Jianwu Wang (University of Maryland Baltimore County, USA), Carl Busart, Robert F.Erbacher (DEVCOM Army Research Laboratory,Adelphi, Maryland, USA)
AbstractCamouflaged object detection is a challenging task in computer vision that involves identifying objects that are intentionally or unintentionally hidden in their surrounding environment. Vision Transformer mechanisms play a critical role in improving the performance of deep learning models by focusing on the most relevant features that help object detection under camouflaged conditions. In this paper, we utilized a vision transformer (VT) in two phases, a) By integrating VT with the deep learning architecture for efficient monocular depth map generation for camouflaged objects and b) By embedding VT in the architecture of the multiclass object detection model with multimodal feature input (RGB with RGB-D) that increases the visual cues and provides more representational information to the model for performance enhancement. Additionally, we performed an ablation study to understand the role of the vision transformer in camouflaged object detection and incorporated GRAD-CAM on top of the model to visualize the performance improvement achieved by embedding the VT in the model architecture. We deployed the model in the resource-constrained edge devices for real-time object detection to realistically test the performance of the trained model.
Elixir: A system to enhance data quality for multiple analytics on a video stream
Authors: Sibendu Paul (Purdue University, USA); Kunal Rao (NEC Laboratories America Inc., USA); Giuseppe Coviello (NEC Laboratories America, Inc., USA); Murugan Sankaradas (NEC Laboratories America Inc., USA); Y. Charlie Hu (Purdue University, USA); Srimat Chakradhar (NEC Research Labs, USA)
AbstractIoT sensors, especially video cameras, are ubiquitously deployed around the world to perform a variety of computer vision tasks in several verticals including retail, health- care, safety and security, transportation, manufacturing, etc. To amortize their high deployment effort and cost, it is desirable to perform multiple video analytics tasks, which we refer to as Analytical Units (AUs), off the video feed coming out of every camera. As AUs typically use deep learning-based AI/ML models, their performance depend on the quality of the input video, and recent work has shown that dynamically adjusting the camera setting exposed by popular network cameras can help improve the quality of the video feed and hence the AU accuracy, in a single AU setting. In this paper, we first show that in a multi-AU setting, changing the camera setting has disproportionate impact on different AUs performance. In particular, the optimal setting for one AU may severely degrade the performance for another AU, and further the impact on different AUs varies as the environmental condition changes. We then present Elixir, a system to enhance the video stream quality for multiple analytics on a video stream. Elixir leverages Multi-Objective Reinforcement Learning (MORL), where the RL agent caters to the objectives from different AUs and adjusts the camera setting to simultaneously enhance the performance of all AUs. To define the multiple objectives in MORL, we develop new AU-specific quality estimator values for each individual AU. We evaluate Elixir through real-world experiments on a testbed with three cameras deployed next to each other (overlooking a large enterprise parking lot) running Elixir and two baseline approaches, respectively. Elixir correctly detects 7.1% (22,068) and 5.0% (15,731) more cars, 94% (551) and 72% (478) more faces, and 670.4% (4975) and 158.6% (3507) more persons than the default-setting and time-sharing approaches, respectively. It also detects 115 license plates, far more than the time-sharing approach (7) and the default setting (0).
Detecting Potholes from Dashboard Camera Images Using Ensemble of Classification Mechanism
Authors: Miku Minami, Hiroo Bekku, Jin Nakazawa and Takafumi Kawasaki (Keio University, Japan)
AbstractRoad damages such as potholes may occur on roads due to aging, which may affect the traffic. Periodic inspections of road damages are difficult due to the high cost of road surveys, and we tend to overlook road damages which is therefore considered to be a problem in a long-term. The development of a system that automatically detects potholes and other road damages from dash cam images can allow inexpensive roadside inspections, and can overall improve the problem of the long-term oversight of road damages. Last year, we conducted a demonstration experiment in Edogawa City, Tokyo, using an existing image-based road damage detection method. From that experiment, we confirmed that the detection of potholes on actual roads often causes false detections due to the presence of shadows and manholes. In this study, we propose a method to reduce false positives in pothole detection which was considered to be a problem through the demonstration experiment, and evaluate its performance. Since we believe that the evaluation based on a pothole-only dataset is not valid, we reconstruct a dataset for evaluation by adding shadow and manhole images for validation. Our method consists of two main components: data expansion by image generation and ensemble of classification mechanisms for object detection models. As a result of the validation on the reconstructed pothole dataset, the average precision (AP), which is a measure to evaluate false positives, was improved by 0.172 compared to the existing method. In addition, the reduction of AR (average Recall), which is a trade-off with AP, was suppressed to 0.07. Since our method is not dependent on the domain of potholes, it is expected to be an effective pipeline in tasks and situations where false positives are more problematic than false negatives due to the high incidence of false positives.
An Online Continuous Semantic Segmentation Framework With Minimal Labeling Effort
Authors: Masud Ahmed and Zahid Hasan (University of Maryland Baltimore County, USA); Tim M Yingling (University of Maryland, Baltimore County, USA); Eric O'Leary (University of Maryland, USA); Sanjay Purushotham (University of Maryland Baltimore County, USA); Suya You (Army Research Laboratory, USA); Nirmalya Roy (University of Maryland Baltimore County, USA)
AbstractThe annotation load for a new dataset has been greatly decreased using domain adaptation based semantic segmentation, which iteratively constructs pseudo labels on unlabeled target data and retrains the network. However, realistic segmentation datasets are often imbalanced, with pseudo-labels tending to favor certain head classes while neglecting other tail classes. This can lead to an inaccurate and noisy mask. To address this issue, we propose a novel hard sample mining strategy for an active domain adaptation based semantic segmentation network, with the aim of automatically selecting a small subset of labeled target data to fine-tune the network. By calculating class-wise entropy, we are able to rank the difficulty level of different samples. We use a fusion of focal loss and regional mutual information loss instead of cross-entropy loss for the domain adaptation based semantic segmentation network. Our entire framework has been implemented in real-time using the Robotics Operating System (ROS) with a server PC and a small Unmanned Ground Vehicle (UGV) known as the ROSbot2.0 Pro. This implementation allows ROSbot2.0 Pro to access any type of data at any time, enabling it to perform a variety of tasks with ease. Our approach has been thoroughly evaluated through a series of extensive experiments, which demonstrate its superior performance compared to existing state-of-the-art methods. Remarkably, by using just 20% of hard samples for fine-tuning, our network has achieved a level of performance that is comparable (≈ 88%) to that of a fully supervised approach, with mIOU scores of 60.51% in the In-house dataset.
Session 5: Networked and Distributed Smart Computing
Wednesday, 15:30 - 16:30
Session Chair: Claudio Cicconetti (IIT-CNR, Italy)
ReplayMPC: A Fast Failure Recovery Protocol for Secure Multiparty Computation Applications using Blockchain
Authors: Oscar G. Bautista and Kemal Akkaya (Florida International University, USA); Soamar Homsi (Air Force Research Laboratory - Information Directorate (AFRL/RI), USA)
AbstractAlthough recent performance improvements to Secure Multiparty Computation (SMPC) made it a practical solution for complex applications such as privacy-preserving machine learning (ML), other characteristics such as robustness are also critical for its practical viability. For instance, since ML training under SMPC may take longer times (e.g., hours or days in many cases), any interruption of the computation will require restarting the process, which results in more delays and waste of computing resources. While one can maintain exchanged SMPC messages in a separate database, their integrity and authenticity should be guaranteed to be able to re-use them later. Therefore, in this paper, we propose ReplayMPC, an efficient failure recovery mechanism for SMPC based on blockchain technology that enables resuming and re-synchronizing SMPC parties after any type of communication or system failures. Our approach allows SMPC parties to save computation state snapshots they use as restoration points during the recovery and then reproduce the last computation rounds by retrieving information from immutable messages stored on a blockchain. Our experiment results on Algorand blockchain show that recovery is much faster than starting the whole process from scratch, saving time, computation, and networking resources.
A Classification Framework for IoT Network Traffic Data for Provisioning 5G Network Slices in Smart Computing Application
Authors: Ziran Min (Vanderbilt University, USA); Swapna S. Gokhale (University of Connecticut, USA); Shashank Shekhar and Charif Mahmoudi (Siemens, USA); Zhuangwei Kang, Yogesh Barve and Aniruddha Gokhale (Vanderbilt University, USA)
AbstractExisting massive deployments of IoT devices in support of smart computing applications across a range of domains must leverage critical features of 5G, such as network slicing, to receive differentiated and reliable services. However, the voluminous, dynamic, and heterogeneous nature of IoT traffic imposes complexities on the problems of network flow classification, network traffic analysis, and accurate quantification of the network requirements, thereby making the provisioning of 5G network slices across the application mix a challenging problem. To address these needs, we propose a novel network traffic classification approach that consists of a pipeline that combines Principal Component Analysis (PCA), with KMeans clustering and Hellinger distance. PCA is applied as the first step to efficiently reduce the dimensionality of features while preserving as much of the original information as possible. This significantly reduces the runtime of KMeans, which is applied as the second step. KMeans, being an unsupervised approach, eliminates the need to label data which can be cumbersome, error-prone, and time-consuming. In the third step, a Hellinger distance-based recursive KMeans algorithm is applied to merge similar clusters toward identifying the optimal number. This makes the final clustering results compact and intuitively interpretable within the context of the problem, while addressing the limitations of traditional KMeans algorithm, such as sensitivity to initialization and the requirement of manual specification of the number of clusters. Evaluation of our approach on a real-world IoT dataset demonstrates that the pipeline can compactly represent the dataset as three clusters. The service properties of these clusters can be easily inferred and directly mapped to different types of slices in the 5G network.
NextGenGW: a Software Framework Based on MQTT and Semantic Data Format
Authors: Carlos Resende and Waldir Moreira (Fraunhofer Portugal AICOS, Portugal); Luis Almeida (Universidade do Porto & Instituto de Telecomunicações, Portugal)
AbstractTo access all the potential value present in IoT, the IoT devices need to be interoperable. Some works in the literature target this issue, but it is not yet entirely solved, mainly because the proposed solutions are not standard based at the semantic level. This paper presents the detailed implementation of our standard-based software framework targeting IoT interoperability, named NextGenGW. In this IoT gateway-based software framework, we propose the first integration of IETF SDF with the MQTT protocol. We define an evaluation baseline for validating IoT gateway performance while focusing on interoperability. Our evaluation results shows the NextGenGW suitability for slow processes, as well as demanding use cases where it needs to be deployed in a device with reduced resources, considering its scalability both in terms of connected IoT end nodes and number of requests per time interval.
Session 6: Machine Learning for Control
Thursday, 10:30 - 12:00
Session Chair: Carlo Vallati(University of Pisa, Italy))
Cooperative Multi-Agent Reinforcement Learning for Large Scale Variable Speed Limit Control
Authors: Yuhang Zhang and Marcos Quinones-Grueiro (Vanderbilt University, USA); William Barbour (Vanderbilt University & Institute for Software Integrated Systems, USA); Zhiyao Zhang and Joshua Scherer (Vanderbilt University, USA); Gautam Biswas and Daniel Work (Vanderbilt University & Institute for Software Integrated Systems, USA)
AbstractVariable speed limit (VSL) control has emerged as a promising traffic management strategy for enhancing safety and mobility. In this study, we introduce a multi-agent reinforcement learning framework for implementing a large-scale VSL system to address recurring congestion in transportation corridors. The VSL control problem is modeled as a Markov game, using only data widely available on freeways. By employing parameter sharing among all VSL agents, the proposed algorithm can efficiently scale to cover extensive corridors. The agents are trained using a reward structure that incorporates adaptability, safety, mobility, and penalty terms; enabling agents to learn a coordinated policy that effectively reduces spatial speed variations while minimizing the impact on mobility. Our findings reveal that the proposed algorithm leads to a significant reduction in speed variation, which holds the potential to reduce incidents. Furthermore, the proposed approach performs satisfactorily under varying traffic demand and compliance rates.
A Novel Weight Dropout Approach to Accelerate the Neural Network Controller Embedded Implementation on FPGA for a Solar Invert
Authors: Jordan Sturtz (North Carolina Agricultural and Technical State University, USA); Xingang Fu (Texas A&M University Kingsville, USA); Chanakya Hingu (Texas A&M University-Kingsville, USA); Letu Qingge (North Carolina Agricultural and Technical State University, USA)
AbstractThis paper introduces a novel weight-dropout approach to train a neural network controller in real-time closed-loop control and to accelerate the embedded implementation for a solar inverter. The essence of the approach is to drop small-magnitude weights of neural network controllers during training with the goal of minimizing the required numbers of connections and guaranteeing the convergence of the neural network controllers. In order not to affect the convergence of neural network controllers, only non-diagonal elements of the neural network weight matrices were dropped. The dropout approach was incorporated into Levenberg-Marquardt and Forward Accumulation Through Time algorithms to train the neural network controller for trajectory tracking more efficiently. The Field Programmable Gate Array (FPGA) implementation on the Intel Cyclone V board shows significant improvement in terms of computation and resource requirements using the sparse weight matrices after dropout, which makes the neural network controller more suitable in an embedded environment.
On learning data-driven models for in-flight drone battery discharge estimation from real data
Authors: Austin C Coursey and Marcos Quinones-Grueiro (Vanderbilt University, USA); Gautam Biswas (Vanderbilt University & Institute for Software Integrated Systems, USA)
AbstractAccurate estimation of the battery state of charge (SOC) of unmanned aerial vehicles (UAV) along a mission is an essential in-flight monitoring task to guarantee the survivability of the system. Physics-based models of the battery have been developed in the past with successful applications. However, in general, these models do not account for the effect of the mission profile and environmental conditions on power consumption. Recently, data-driven methods have been leveraged given their ease-of-use and scalability. Yet, most benchmarking experiments have been conducted on simulated battery datasets. In this work, we compare different data-driven models for battery SOC estimation of a hexacopter UAVs by using real flight data. We analyze the importance of numerous flight variables under different environmental conditions to determine which factors impact battery consumption over the course of the flight. We demonstrate that additional flight variables are necessary to create an accurate SOC estimation model through data-driven methods.
E-ADDA: Unsupervised Adversarial Domain Adaptation Enhanced by a New Mahalanobis Distance Loss for Smart Computing
Authors: Ye Gao (University of Virginia, USA); Brian Baucom (University of Utah, USA); Karen Rose (Ohio State University, USA); Kristina Gordon (University of Tennessee, USA); Hongning Wang and John Stankovic (University of Virginia, USA)
AbstractIn smart computing, the labels of training samples for a specific task are not always abundant. However, the labels of samples in a relevant but different dataset are available. As a result, researchers have relied on unsupervised domain adaptation to leverage the labels in a dataset (the source domain) to perform better classification in a different, unlabeled dataset (target domain). Existing non-generative adversarial solutions for UDA aim at achieving domain confusion through adversarial training. The ideal scenario is that perfect domain confusion is achieved, but this is not guaranteed to be true. To further enforce domain confusion on top of the adversarial training, we propose a novel UDA algorithm, E-ADD A, which uses both a novel variation of the Mahalanobis distance loss and an out-of-distribution detection subroutine. The Mahalanobis distance loss minimizes the distribution-wise distance between the encoded target samples and the distribution of the source domain, thus enforcing additional domain confusion on top of adversarial training. Then, the OOD subroutine further eliminates samples on which the domain confusion is unsuccessful. We have performed extensive and comprehensive evaluations of E-ADDA in the acoustic and computer vision modalities. In the acoustic modality, E-ADDA outperforms several state-of-the-art UDA algorithms by up to 29.8%, measured in the f1 score. In the computer vision modality, the evaluation results suggest that we achieve new state-of-the-art performance on popular UDA benchmarks such as Office-31 and Office-Home, outperforming the second best-performing algorithms by up to 17.9%.
Demos/Posters
DEMOS
A Demo of Microservice for Customized Faulty Product Detection System in Smart Manufacturing
Nitesh Bharot, John G. Breslin and Mirco Soderi (University of Galway, Ireland)
Real-World Community-in-the-Loop Smart Video Surveillance System
Shanle Yao, Babak Rahimi Ardabili, Armin Danesh Pazho, Ghazal Alinezhad Noghre, Christopher Neff and Hamed Tabkhi (University of North Carolina at Charlotte, USA)
Distributed Control Application for Smart Grids using RIAPS
Purboday Ghosh, Niloy Barua and Timothy Krentz (Vanderbilt University, USA); Gabor Karsai (Vanderbilt University/ISIS, USA); Abhishek Dubey (Vanderbilt University, USA); Srdjan Lukic (North Carolina State University, USA)
A prototype for QKD-secure Serverless Computing with ETSI MEC
Claudio Cicconetti and Marco Conti (IIT-CNR, Italy); Eufemia Lella and Pietro Noviello (Exprivia SpA, Italy); Gennaro Davide Paduanelli (Exprivia, Italy); Andrea Passarella (IIT-CNR, Italy); Elisabetta Storelli (Exprivia S.p.A., Italy)
Microtransit Optimizer for Mobility-on-Demand
Michael Wilbur, Sophie Pavia, Abhishek Dubey, Pravesh Koirala, and Zakariyya Al-Quran (Vanderbilt University, USA); Maxime R Coursey (3436 Briarcliff Rd NE & Vanderbilt University, USA); Philip Pugliese (Chattanooga Area Regional Transportation Authority, USA)
POSTERS
A Case Study Using Zoom Touch Gestures: How Does the Size of a Training Dataset Impact User's Age Estimation Accuracy in Smartphones?
Md Hossain (Southern Connecticut State University, USA)
A Service for Resilient Manufacturing
Mirco Soderi (National University of Ireland, Galway, Ireland); John G. Breslin (University of Galway, Ireland)
Advancing Technology-Enabled Mobility Solutions in Tennessee
Kevin Heaslip (University of Tennessee, Knoxville, USA)
Cyber Framework for Steering and Measurements Collection Over Instrument-Computing Ecosystems
Anees Al-Najjar, Nageswara Rao, Ramanan Sankaran, Helia Zandi, Debangshu Mukherjee, Maxim Ziatdinov and Craig Bridges (Oak Ridge National Laboratory, USA)
Cyberattack Detection, Mitigation, and Best Practices Guidance in a Connected and Automated Vehicle Intersection
Kevin Heaslip (University of Tennessee, Knoxville, USA)
A Data Driven Risk Assessment of Arctic Maritime Incidents: Using Machine Learning to Predict Incident Types and Identify Risk Factors
Rajesh Kandel and Hiba Baroud (Vanderbilt University, USA)
Cooperative Multi-Agent Reinforcement Learning for Large Scale Variable Speed Limit Control
Yuhang Zhang and Marcos Quinones-Grueiro (Vanderbilt University, USA); William Barbour (Vanderbilt University & Institute for Software Integrated Systems, USA); Zhiyao Zhang and Joshua Scherer (Vanderbilt University, USA); Gautam Biswas and Daniel Work (Vanderbilt University & Institute for Software Integrated Systems, USA)
DQN for Smart Transportation Supporting V2V Mobile Edge Computing
Xiaoming Guo (The University of Alabama, USA); Xiaoyan Hong (University of Alabama, USA)
KissLoc - A Spatio-temporal Kissing Recognition System Using Commercial Smart Glasses
Hamada Rizk and Hirozumi Yamaguchi (Osaka University, Japan)
Nisshash: Design of An IoT-based Smart T-Shirt for Guided Breathing Exercises
Md Abdullah Al Rumon and Kunal Mankodiya (University of Rhode Island, USA)
Improving Product Quality Control in Smart Manufacturing through Transfer Learning-Based Fault Detection
Nitesh Bharot (University of Galway, Ireland); Mirco Soderi (National University of Ireland, Galway, Ireland); Priyanka Verma and John G. Breslin (University of Galway, Ireland)
Microtransit Optimizer for Mobility-on-Demand
Michael Wilbur, Sophie Pavia, Abhishek Dubey, Pravesh Koirala, and Zakariyya Al-Quran (Vanderbilt University, USA); Maxime R Coursey (3436 Briarcliff Rd NE & Vanderbilt University, USA); Philip Pugliese (Chattanooga Area Regional Transportation Authority, USA)
Using Innovations in Data Analytics and Smart Technologies to Fight Opioid Overdose Crisis
Nasibeh Zohrabi (Pennsylvania State University, USA); Jacqueline B. Britz and Alex H. Krist (Virginia Commonwealth University, USA); Mostafa Zaman (VIrginia Commonwealth University, USA); Sherif Abdelwahed (Virginia Commonwealth University, USA)
Performance Tradeoff in DNN-based Coexisting Applications in Resource-Constrained Cyber-Physical Systems
Elijah Spicer and Sabur Baidya (University of Louisville, USA)
Knowledge-embedded Prompt Learning for Zero-shot Social Media Text Classification
Jingyi Li (XJTLU, China); Qi Chen and Wei Wang (Xi'an Jiaotong Liverpool University, China); Fangyu Wu (XJTLU, China)
Single Camera-enabled Reinforcement Learning Traffic Signal Control System supporting Life-long Assessment
Toan Tran (University of Tennessee at Chattanooga, USA); Mina Sartipi (University of TN at Chattanooga, USA)
Synchronized Sub-Second Arbitrary Changes to Decoupled Components for Ultimate Resilience in Cross-Platform Geo-Distributed Smart Factories
Mirco Soderi (National University of Ireland, Galway, Ireland); John G. Breslin (University of Galway, Ireland)
Robust Detection of Social Isolation in Older Adults by Combining Biometrics with Social Interaction Data
Raghav S Mehrotra-Venkat (University High School, USA); Nikil Dutt (University of California, Irvine, USA); Julie Rousseau (University of California Irvine School of Medicine, USA)
FedTIU: Securing Virtualized PLCs Against DDoS Attacks Using a Federated Learning Enabled Threat Intelligence Unit
Priyanka Verma (University of Galway, Ireland); Miguel Ponce de Leon (VMware, Ireland); John G. Breslin (University of Galway, Ireland); Donna OShea (Munster Technological University, Ireland)
WiP Presentations
In this session, each paper is given 5 minutes to present their work in a presentation style format.
A Case Study Using Zoom Touch Gestures: How Does the Size of a Training Dataset Impact User's Age Estimation Accuracy in Smartphones?
Md Hossain (Southern Connecticut State University, USA)
Cyber Framework for Steering and Measurements Collection Over Instrument-Computing Ecosystems
Anees Al-Najjar, Nageswara Rao, Ramanan Sankaran, Helia Zandi, Debangshu Mukherjee, Maxim Ziatdinov and Craig Bridges (Oak Ridge National Laboratory, USA)
Improving Product Quality Control in Smart Manufacturing through Transfer Learning-Based Fault Detection
Nitesh Bharot (University of Galway, Ireland); Mirco Soderi (National University of Ireland, Galway, Ireland); Priyanka Verma and John G. Breslin (University of Galway, Ireland)
GNN-RL: Dynamic Reward Mechanism for Connected Vehicle Security using Graph Neural Networks and Reinforcement Learning
Heena Rathore (Texas State University, USA); Henry Griffith (San Antonio College, USA)
Using Innovations in Data Analytics and Smart Technologies to Fight Opioid Overdose Crisis
Nasibeh Zohrabi (Pennsylvania State University, USA); Jacqueline B. Britz and Alex H. Krist (Virginia Commonwealth University, USA); Mostafa Zaman (VIrginia Commonwealth University, USA); Sherif Abdelwahed (Virginia Commonwealth University, USA)
Performance Tradeoff in DNN-based Coexisting Applications in Resource-Constrained Cyber-Physical Systems
Elijah Spicer and Sabur Baidya (University of Louisville, USA)
Knowledge-embedded Prompt Learning for Zero-shot Social Media Text Classification
Jingyi Li (XJTLU, China); Qi Chen and Wei Wang (Xi'an Jiaotong Liverpool University, China); Fangyu Wu (XJTLU, China)
Single Camera-enabled Reinforcement Learning Traffic Signal Control System supporting Life-long Assessment
Toan Tran (University of Tennessee at Chattanooga, USA); Mina Sartipi (University of TN at Chattanooga, USA)
Synchronized Sub-Second Arbitrary Changes to Decoupled Components for Ultimate Resilience in Cross-Platform Geo-Distributed Smart Factories
Mirco Soderi (National University of Ireland, Galway, Ireland); John G. Breslin (University of Galway, Ireland)
Robust Detection of Social Isolation in Older Adults by Combining Biometrics with Social Interaction Data
Raghav S Mehrotra-Venkat (University High School, USA); Nikil Dutt (University of California, Irvine, USA); Julie Rousseau (University of California Irvine School of Medicine, USA)
FedTIU: Securing Virtualized PLCs Against DDoS Attacks Using a Federated Learning Enabled Threat Intelligence Unit
Priyanka Verma (University of Galway, Ireland); Miguel Ponce de Leon (VMware, Ireland); John G. Breslin (University of Galway, Ireland); Donna OShea (Munster Technological University, Ireland)
Optimization and Improvement of Fake News Detection using Voting Technique for Societal Benefit
Sneha Karki, Zichong Wang and Tyler Zetty (Michigan Technological University, USA); Tongjia Yu (Columbia University in the City of New York, USA); Puqing Jiang (Huazhong University of Science and Technology, China); Wenbin Zhang (University of Maryland, Baltimore County, USA)
Improving Reinforcement Learning Performance through a Behavioral Psychology-Inspired Variable Reward Scheme
Heena Rathore (Texas State University, USA); Henry Griffith (San Antonio College, USA)
PhD Forum
1570908270
Efficient 3D Feature Learning for Real-Time Awareness
Authors: Ta-Ying Cheng (University of Oxford, United Kingdom (Great Britain))
Abstract: This extended abstract discusses the current methods and work progress on sampling large-scale point cloud datasets with semantics and reconstructing 3D objects from sparse inputs. In particular, we describe a proposed meta sampling strategy to quickly adapt sampling to multiple tasks and potential methods to improve multi-modal reconstruction. These methods could benefit immensely in creating in-depth situational awareness for challenging missions and rescues.
1570908733
Multi-modal AI Systems for Human and Animal Pose Estimation in Challenging Conditions
Authors: Qianyi Deng (University of Oxford, United Kingdom (Great Britain))
Abstract: This paper explores the development of multi-modal AI systems for pose estimation in challenging conditions for both humans and animals. Existing single-modality approaches struggle in challenging scenarios such as emergency response and wildlife observation due to factors like smoke, low light, obstacles, and long-distance observations. To address these challenges, this research proposes integrating multiple sensor modalities and leveraging the strengths of different sensors to enhance accuracy and robustness in pose estimation.
1570908803
Investigating Computational Aspects and Potential Challenges in Implementing Urban Air Mobility
Authors: Debjyoti Sengupta (Missouri University of Science and Technology, USA)
Abstract:Urban Air Mobility (UAM) involving piloted or autonomous aerial vehicles is envisioned as an emerging disruptive technology for next generation smart transportation that addresses mobility challenges in congested cities. This paradigm may include aircrafts ranging from small unmanned aerial vehicles (UAVs) or drones, to aircrafts with passenger carrying capacity, such as personal air vehicles (PAVs). This paper highlights the UAM vision and brings out the underlying fundamental research challenges and opportunities from computing, networking, and service perspectives for sustainable design and implementation of this promising technology providing an innovative infrastructure for urban mobility. Important research questions include, but are not limited to, real-time autonomous scheduling, dynamic route planning, aerial-to-ground and inter-vehicle communications, airspace traffic management, on-demand air mobility, resource management, quality of service and quality of experience, sensing (edge) analytics and machine learning for trustworthy decision making, optimization of operational services, and socio-economic impacts of UAM infrastructure on sustainability.
1570909224
Nisshash: Design of An IoT-based Smart T-Shirt for Guided Breathing Exercises
Authors: Md Abdullah Al Rumon (University of Rhode Island, USA)
Abstract:Breathing exercises are gaining attention in managing anxiety and stress in daily life. Diaphragmatic breathing, in particular, fosters tranquility for both body and mind. Existing use cases, such as meditation, yoga, and medical devices for guided breathing, often require expert guidance, complex instruments, cumbersome devices, and sticky electrodes. To address these challenges, we present Nisshash, an IoT-based smart T-shirt offering a personalized solution for regulated breathing exercises. Nisshash is embedded with three-channel e-textile respiration sensors and a tailored analog front-end (AFE) board to monitor respiration rate (RR) and heart rate (HR) simultaneously. In this work, we seamlessly integrate soft textile sensors into a T-shirt and develop a detachable and Wi-Fi-enabled (2.4GHz) bio-instrumentation board, creating a pervasive wireless system (WPS) for guided breathing exercises (GBE). The system features an intuitive graphical user interface (GUI) and a seamless IoT-based control and computing system (CCS). It offers real-time instructions for inhaling and exhaling at various breathing speeds, including slow, normal, and fast breathing. We conducted a study with 10 healthy adult participants who wore the T-shirt and performed guided breathing exercises. The average respiration event (inhale-exhale) detection accuracy was ≈ 98%. We validated the recorded HR against the 3-lead PC-80B ECG monitoring device, achieving an accuracy of ≈ 99%. The RRHR correlation analysis showed an R square value of 0.987. Collectively, these results demonstrate Nisshash's potential as a personal guided breathing exercise solution.
1570909239
Detecting False Data Injection in a Large-Scale Water Distribution Network
Authors: Ayanfeoluwa Oluyomi (Missouri University of Science and Technology, USA)
Abstract: Utility companies rely on accurate data (which can be energy or water consumption) to monitor and manage the pricing, and distribution of resources and in this context, determine peak periods of water consumption throughout the year. In most cities, a utility company tends to service a large number of houses in that city. Thus, making it difficult for them to manage an attack because of the different patterns that exist in various neighborhoods. As a result of this, an adversary can compromise the meters by injecting false data. False data injection (FDI) attacks to compromise the integrity of the data, leading to inaccurate decision-making and potential water resource wastage. To address this problem, this research aims to study a clustering algorithm that leverages graph theory to cluster houses with similar water usage patterns in a city. After this, an FDI detection model is run on each cluster to identify any attack.
1570909305
System Modeling and Co-Emulation for Distributed Cyber-Physical System Environments
Authors: Nathan A Puryear (Virginia Commonwealth University, USA)
Abstract: This paper presents work in progress towards a system modeling and co-emulation framework for distributed cyber-physical system (CPS) environments. The proposed framework aims to support experiential learning and experiment orchestration in environments such as CPS testbeds and chemistry labs. It addresses challenges of interoperability, multi-tenancy, scalability and security by leveraging a novel co-emulation approach that combines different modeling, orchestration and runtime tools.
1570909346
DOT - Digital Orchestration of Things
Authors: Carlos Resende (Fraunhofer Portugal AICOS, Portugal)
Abstract: The number of IoT device connections is growing at a sustained pace, potentiating the rise of new business models, such as sensing as a service. However, the current provisioning and life cycle management of IoT end nodes (the devices that sense and interact with the environment) based on pre-configured/pre-installed software and manual updating procedures cannot sustain this continuous growth and the new service-oriented business models. We propose the DOT to solve this problem. DOT automates IoT end nodes' provisioning and life cycle management using standards to avoid vendor lock-in.
1570909534
A Model Based Decision Support System for Smart Cities
Authors: Mostafa Zaman (VIrginia Commonwealth University, USA)
Abstract: The escalating population growth and urbanization have led to a surge in the demand for smart cities. Nonetheless, handling and evaluating the vast data produced by Internet of Things (IoT) sensors requires significant effort. Therefore, implementing intelligent decision support systems is crucial for analyzing real-time data and optimizing city operations while tackling uncertain events. This study discusses the architectural flow diagram of a smart city decision support system that employs reinforcement learning techniques to enhance traffic management, minimize energy consumption, elevate public safety, and reduce risks in a constantly changing and unpredictable environment. This system comprises various components that work in tandem to provide customized real-time recommendations for a given situation. The capacity of the system to produce recommendations in real-time while taking into account the likelihood of various outcomes has the potential to enhance performance and facilitate more efficient decision-making in intricate settings. In general, this system will exhibit the capability to improve emergency response and public safety to a considerable extent in smart cities.
1570909690
Traffic Routing under Driver Distrust
Authors: Doris E M Brown (Missouri University of Science and Technology, USA)
Abstract: Traditional strategic information design literature assumes receivers trust the signals shared by the sender, the sender and receivers have symmetric information at the outset of the interaction, and receivers update their beliefs according to Bayes rule. In our work, we consider an interaction between a strategic central routing system and multiple drivers as a Stackelberg game within a traffic network in which the leader may perturb traffic information shared with selfish receivers to reach a system-optimal routing outcome that minimizes network congestion. We propose a framework that deviates from the traditional assumptions of the strategic information design framework to better mimic real-world human behavior and consider conditions under which a sender shares deceptive information with a receiver.
1570910911
Privacy-preserving Real-world Video Anomaly Detection
Authors: Ghazal Alinezhad Noghre (University of North Carolina at Charlotte, USA)
Abstract: Video anomaly detection is a significant problem in computer vision that aims to detect unusual or abnormal behaviors in video data that can be used to enhance public safety. Given the widespread deployment of cameras in public areas, video anomaly detection for public safety has become increasingly important in recent years. There are numerous applications, including but not limited to security, traffic monitoring, healthcare, and manufacturing, where video anomaly detection can be useful. However, anomaly detection in nature is an open-set problem that further complicates the task. Moreover, the definition of anomalous behavior may differ in various environments, adding to real-world anomaly detection challenges. On the other hand, addressing ethical issues and privacy concerns related to this task is also crucial. We aim to design an anomaly detection method that uses non-identifiable features such as pose, trajectory, and optical flow to avoid discrimination against distinct minority groups and safeguard the privacy of individuals.